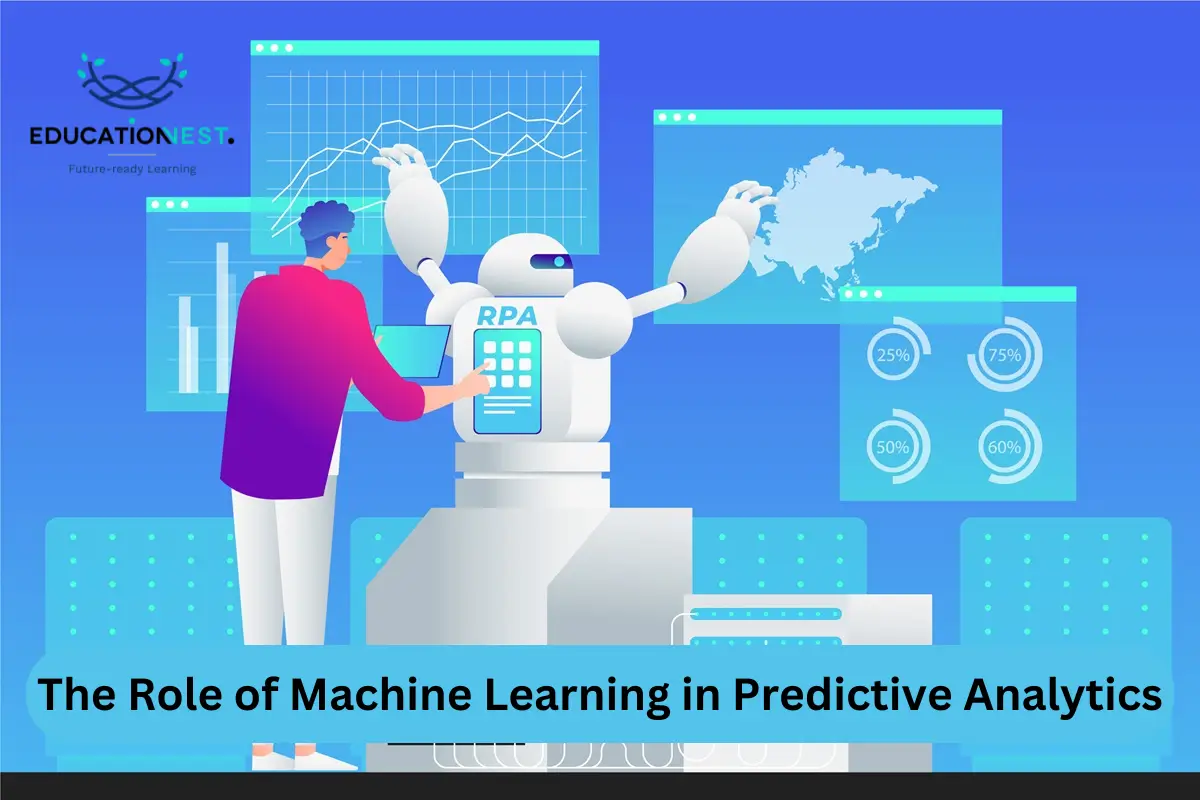
Imagine if you were granted the superpower to predict the next bull run in the stock market. This is not far in our future – especially as we now coexist with AI and ML. This reality is made possible by predictive analytics powered by machine learning. With ML, businesses can now move from reactive to proactive decision-making. Predictive analytics is all about using advanced data techniques to gain deep insights for predicting the future. The process is quite complex involving predictive modeling, data mining, ML, and neural networks. In this article, we explore the role of ML in predictive analytics and how it is helping businesses make better decisions.
Foundations of Machine Learning
In this section, let us learn the basics of machine learning. From the basic definition to the different types of ML, we have to know the foundational things first to grasp its role in predicting trends.
Explaining Machine Learning
Machine learning is the driving factor behind the growth of predictive analytics. At its core, ML enables computer systems to learn from data and improve their performance as they get access to more data over time. They enable computers to do this without needing any specific instructions from humans. It is like teaching a computer to learn from past information.
Supervised Learning
This method uses labeled data. This means each example in the dataset comes with a known outcome. The model is trained on this data so that it can predict outcomes for new data. Take this scenario as an example:
To predict house prices, the model will first need to read through a dataset containing house prices segregated according to their features (size, location, number of rooms). It will eventually learn from these examples and get better at predicting prices for similar houses.
Unsupervised Learning
Unlike supervised learning, this method deals with data that does not have any labeled outcomes. The model will try to find patterns in this data without any guidance. It is the same as trying to explore a new city without a map. You will start to notice landmarks and make sense of the layout over time. A common use of unsupervised learning is customer segmentation.
Benefits of Machine Learning in Predictive Analytics
Integrating ML into predictive analytics offers many benefits which can greatly change forecast outcomes. ML can offer deeper insights for businesses than traditional data analysis can. Here is a closer look at some of the key advantages of machine learning in predictive analytics:
- Scalability
To gain deeper insight, we need to be able to analyze large datasets efficiently. ML is well-suited for this challenge as it can process vast amounts of data quickly. This means businesses can keep up with the increasing data volume without compromising on analysis speed.
- Enhanced Accuracy
One of the biggest advantages of using machine learning in predictive analytics is the improved accuracy of predictions. ML algorithms are designed to identify subtle patterns and relationships within complex data sets. For highly precise forecasts, it is the subtle details that matter.
- Real-time Analysis
ML models are capable of processing data as it comes in. This becomes even more important in industries where you need to make rapid decisions. This could be sectors like finance, e-commerce, or supply chain management.
- Complex Relationship Modeling
ML excels at finding complex relationships between variables that traditional methods often struggle with. This is another benefit of ML that it can identify subtle relations between variables that might not be obvious at first. Such a deep understanding of data allows businesses to find the insights that bring in the most profits.
- Customization and Personalization
ML lets businesses create predictive models that can even be tailored to specific needs. This is particularly valuable in the marketing industry where personalized campaigns have the best results and conversion rates.
- Continuous Learning
Traditional models remain static after being built. But, a major advantage of ML models is that they keep learning and adapting as they receive new data. This allows them to stay up-to-date with changes in trends and behaviors. As you can guess, this is extremely crucial for their predictions to remain relevant.
- Prescriptive Analytics
ML not only helps predict the future but can also provide prescriptive insights. This means that it can suggest actionable steps that will help achieve the desired results. For example, a logistics company might use ML to not only predict delivery delays but also get suggestions on how to reroute shipments to avoid those delays.
- Cost Efficiency
While building and training machine learning models require initial investments, they can significantly reduce operational costs in the long run. For example, predictive maintenance models are now being used to analyze machine data to anticipate when the equipment is likely to fail. This allows businesses to schedule maintenance slots before breakdowns occur. This minimizes downtime and repair costs.
Because businesses are starting to recognize the immense potential of this field, this is the right time to enter this field. If your company is shifting towards AI and ML projects, it might be worthwhile to train your workforce with the necessary skills in this domain. If you are looking for comprehensive big data courses for corporate teams, EducationNest, India’s leading corporate training provider offers expert-led training programs to help companies build their in-house big data analytics teams.
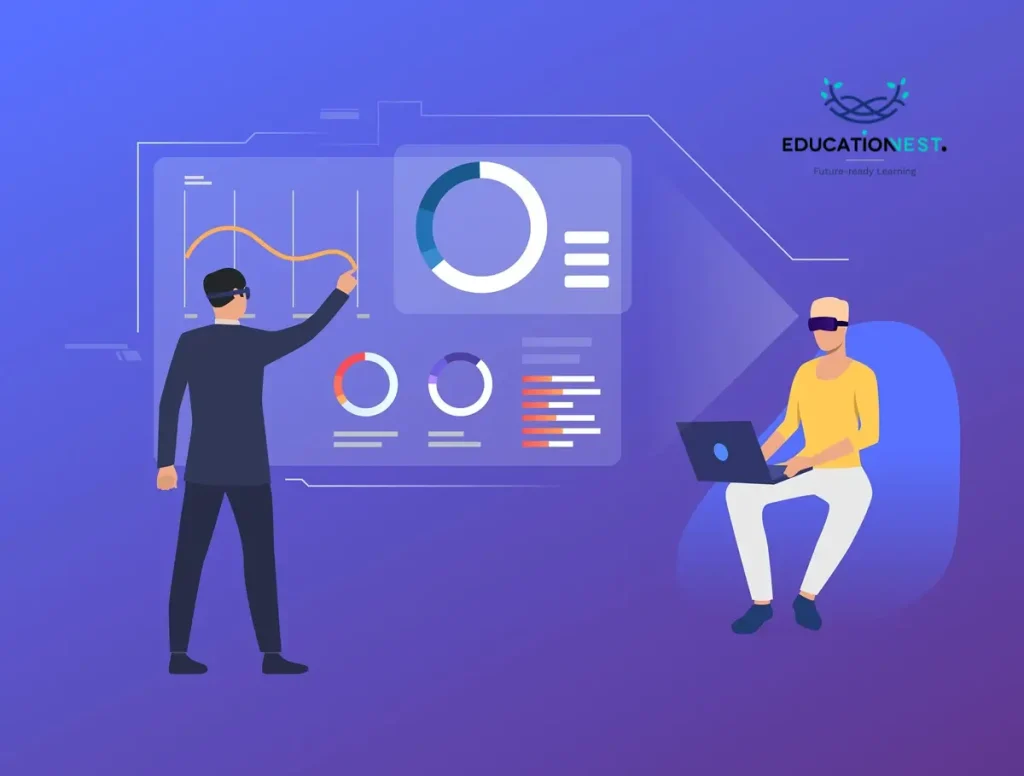
Read More
Time Blocking vs. To-Do Lists: Which Time Management Technique Works Best?
Power BI vs. Excel: When Should You Use Each for Data Analysis?
Key Machine Learning Techniques in Predictive Analytics
If you are into building predictive models, there are a lot of ML techniques for predictive analytics that are used today. From the basics like regression analysis to clustering, here are some of the most common and important ones you should know:
- Regression Analysis
This is an important machine-learning technique used to understand how different factors are linked to one another. It is often used in prediction models for sales, temperature/weather changes, and market trends. For example, as a real estate company, you might use regression to predict house prices based on factors like location, size, and market conditions.
- Classification Models
Classification models are useful when the goal is to categorize data into specific groups. They are widely used in scenarios like the detection of email spam, fraud, and medical diagnosis. A bank might use this machine-learning technique to identify whether a transaction is likely fraudulent based on past transaction history. These models learn from historical data to make accurate predictions about new data.
- Clustering
This technique used in predictive analytics helps in grouping similar data points together. It is most commonly used for exploratory analysis and customer segmentation. You will often find this technique being used in the retail world. Retail companies used this to group customers based on purchasing behavior to create targeted marketing campaigns.
- Neural Networks
This is a type of ML model inspired by the human brain. Neural networks are pros in working with complex, non-linear relationships in data. They consist of layers of interconnected nodes, each of which processes a small piece of information. They are especially valuable for tasks like image and speech recognition, and predicting complex financial trends.
Conclusion
Predicting the future is probably one common thing that excites everyone equally. If only there was a way to predict when the stock markets will run upside next or which company will find the cure to the deadliest cancers. Well, if not all of them, ML is helping us answer some of our questions about the future through predictive analytics. You just need to know how to work with these models to predict the next best thing that could make your business the next Amazon!
If you are looking to build your own in-house teams of the best machine learning experts, EducationNest has the best big data courses for your corporate teams! Offering numerous courses spread over various fields, their expert-led courses have a proven track record of training the best minds in the corporate world.