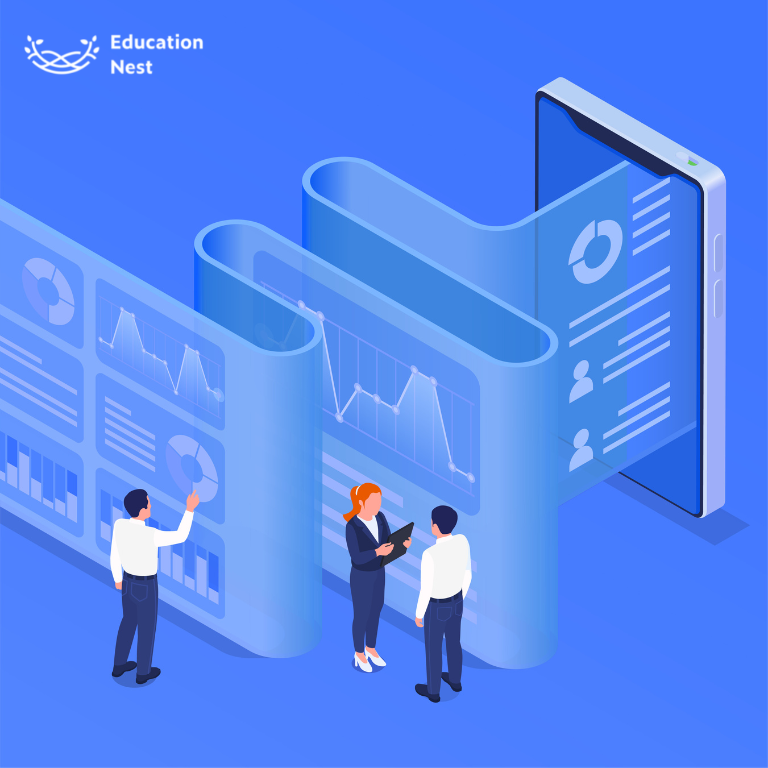
Data has become an essential resource in today’s world. In every aspect of our lives, we are generating, processing, and analyzing large amounts of data but utilizing the data correctly is the most important thing for everybody. Analyzing the data with the correct tools and choosing the right tools is the most challenging question. Data analytics plays an essential role in today’s world by helping businesses and organizations to make informed decisions based on data-driven insights. This blog post will discuss a data analytics tool list for beginners and top data analytics tools for research and open source data analytical tools.
What do you mean by data analytics tool?
Data analytical tools are programmes and software that collect, gather, and analyse data about a business, its customers, and its competitors . This helps a business get an edge over its competitors, improve its processes, and find the insights it needs to make decisions based on data. Most of the time, these tools have different features for managing data, visualising data, analysing data statistically, and teaching machines to learn. They can work with both structured and unstructured data from other sources, such as databases, files, or web services.
How to choose a data analysis tool?
Choosing the correct data analytical tool might be a difficult task for you because so many options exist. Consider these factors while selecting a tool for data analysis:
- Your goals and objectives: When choosing a tool for analyzing data, it’s essential to put your needs in order of importance. Why are you doing this analysis, and what kind of information are you looking at? Do you need the help of an expert in data visualization, statistics, or making predictions? If you know what you want, you can choose the tool to help you do it.
- Easy to use: One of the most important things about a tool for analyzing data is how easy it is to use. If you’re starting in data analysis, it’s best to go with something that doesn’t have a steep learning curve. On the other hand, an experienced data analyst may prefer a more complex tool with more features and configuration options.
- Cost: The fact that price of a data analytical tool can be different from others due to a lot of results . Some tools are free or less expensive , but other can cost a lot. It’s essential to think about the money you have and how much the product will help your business for decision making .
- Data sources and integration: You should also consider the data sources you’ll use and whether they will work with your chosen tool. Choose a program that can easily connect to all of your data sources and give you an overall view of everything you have.
- Scalability: Another essential thing to think about is scalability. If you are working with massive datasets or need to do complex analytics, choosing a tool that can grow with your needs is necessary.
When choosing a data analysis tool, you must think carefully about your business needs, data sources and how they work together, scalability, pricing, support, documentation, and other factors. When you think about all of these things together, you’ll be able to find the data analysis tool that will work best for you.
Data analytics tool for beginners:
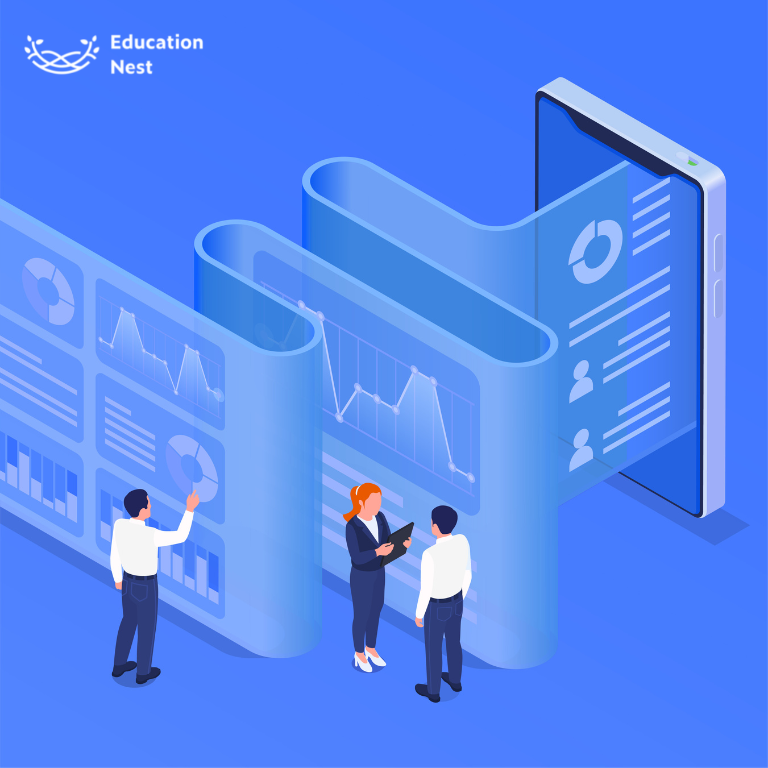
1. Google Analytics: Google Analytics is one of the most popular web analytics services regarding traffic, user activity, and marketing return on investment. Google Analytics is a popular tool for looking at how people use websites and how people use them.
For Google Analytics to work, a tracking code must be put on every website page. With this tracking code, we can find out where our visitors are coming from, what devices they’re using to get to the site, and how they use the area while they’re there. Google Analytics gets the data, processes it, and shows the results in several dashboards and reports.
The main benefit of Google Analytics is the information it gives website owners about who visits their site and how they use it. This can show the people who run a website how people find it, what content is most popular, and what pages make people leave. Once this information is collected, we can improve the website’s structure, content, and overall user experience.
2. MonkeyLearn: monkeylearn is a no-code machine learning platform that provides entire room of advanced data analytical tools to analyze unstructured data around the clock. The pre-trained models in MonkeyLearn can be used immediately to analyze large data sets automatically, or you can use the easy-to-use interface to train your models to analyze text.
Classifying data by topic , sentiment, intent, and more with MonkeyLearn’s data analytical tools and extracting critical information like names, places, and keywords are the tip of the iceberg. The native integration and powerful API of the platform make it easy to connect to the machine-learning tools on MonkeyLearn.
MonkeyLearn Studio has a tool for data visualization, making it easy to move from data analysis to data visualization in one step.
3.RapidMiner: RapidMiner is an enterprise level data science platform for making machine learning models for predictive analytics and implementing them . You’ll need a technical background because it’s made for data analytics teams that want to do challenging jobs and handle a lot of data.
Some solutions are TurboPrep , which helps you clean and prepare your data, AutoModel, which gives you different ways to build machine learning models; and DataStudio, which lets you set up a visual workflow and study your data .
4. Microsoft Excel: Microsoft Excel is the best tool for fundamental data analytics because it can filter , organize, and visualize numerical data. You can use different algorithms and filters and make pivot tables, charts, and graphs to make sense of your data. But Excel has limits, and you may need more powerful tools to analyze a lot of data.
Excel is a powerful program for getting helpful information from big data sets. But it can also do simple math and track almost any information. All of this is possible because of the grid of cells. Cells can store data differently, like text, numbers, or formulas.
5. Airtable: Airtable says its easy-to-use cloud collaboration app is “part spreadsheet, part database.” Like other spreadsheet programs, it can analyze and show data but also has a robust database that gives it extra features. Thanks to “views,” which make it easy to manage, track, and find data in a database, it’s easy to control, track, and see data in a database. There is also an API that lets it work with Airtable and other programs.
6. KNIME can standardize data from many different spreadsheets, flat files, databases, and other sources for direct marketing. These numbers can be normalized, evaluated, and set up in many ways to make graphs. But can use it to create knowledge out of raw data. Because these pieces of information are all in one place, they can make helpful charts and graphs.
Adding KNIME to their marketing technology stack is an excellent way for direct marketers to make sense of the vast amounts of data their operations generate.
Right now, you can use a lot of BI tools that have already been set up. Data visualization tools aren’t just for making great apps; they can also create a handy dashboard for analyzing direct marketing data with the correct settings.
7. Apache Spark: Hadoop MapReduce is a set of algorithms for processing large data sets in parallel and different places. Programmers can make highly parallelized operators without worrying about load balancing or possible failures. MapReduce has a problem because a job has to be run in a series of steps. At each stage, MapReduce gets data from the cluster, processes it, and stores the results in HDFS. MapReduce jobs take longer to finish because disc I/O takes longer at each stage.
Spark was made to fix its flaws by processing in memory, shortening the number of steps needed for a job, and sharing data between many operations going on at the same time. Spark’s simplified way of reading data into memory, processing it, and writing back the results makes iterations much faster. Machine learning algorithms that often run the same function on the same dataset can benefit significantly from Spark’s ability to reuse data by storing it in a memory cache. DataFrames are built on the Resilient Distributed Dataset (RDD), a group of objects cached in memory to use data in multiple Spark computations. In particular, this makes Spark several times faster than MapReduce regarding machine learning and interactive analytics, as the latency is significantly reduced.
8. Knime: This powerful, free, open-source data mining tool with a drag-and-drop interface that helps data scientists build apps and services that stand on their own. It works well as a source of business intelligence and can be used in data analytics. This program is free to download from their website.
You Must Watch: The Ultimate Guide to Understanding the Various Types of Artificial Intelligence
Top Data Analytical Tools:
1)Tableau: Tableau is one of the most popular BI tools because it makes looking at and visualizing data easy. Getting on the list of 2020 leaders in Gartner’s Magic Quadrant For the seventh year in a row, Tableau has made it possible for users to work with real-time data sets and spend more time on Data Analysis and less time on Data Wrangling. Tableau Product Family includes the following:
• Tableau Desktop
• Tableau Server
• Tableau Online
• Tableau Reader
• Tableau Public
If you want to use Tableau to make visualizations but don’t want to pay for the software, you can instead use Tableau Public. To do this, upload your workbook or worksheets to Tableau Server. Then, anyone who has access to that server will be able to use them.
2. Python: Python is a popular programming language for data analytics due to its simplicity, flexibility, and robust data manipulation and analysis libraries.
Python has several powerful data analytics tools, making it popular among data analysts and scientists. Here are some of the most commonly used Python data analytics tools:
- Pandas: The Pandas data structure library is a popular open-source tool for manipulating and analyzing data. It has features that make it easy to work with and analyze data.
- NumPy: NumPy is another well-known Python package for scientific computing. Several tools make combining C/C++ and Fortran code and an N-dimensional solid array object easy.
- Matplotlib: Matplotlib is a Python library for making visualizations. Many people use it because it has many options for making static and moving representations. It is also often used to create 2D and 3D plots, histograms, and scatterplots.
- Scikit-learn: Scikit-learn is a popular machine-learning library in Python that provides a range of algorithms for regression, classification, clustering, and dimensionality reduction. It also provides tools for model selection and evaluation.
- TensorFlow: Google’s TensorFlow is a machine learning library that is free and open-source. It can build and train deep learning models, such as convolutional and recurrent neural networks.
- Keras: Keras is an API for neural networks in Python with a high abstraction level. Keras makes it easy to make and train deep-learning models. It is based on TensorFlow and has a simple interface that makes it easy to make and teach neural networks.
3. R: is a popular open-source statistical computing and data analysis programming language. Data analysts and scientists often use it because it has several powerful tools for analyzing data. Here are some of the most used tools for analyzing data in
RStudio is a popular integrated development environment (IDE) for R. It has a simple interface for writing R code and many tools for analyzing data, such as a data viewer, a code editor, and a console.
- ggplot2: People often use the R library ggplot2 to plot data. This strong graphical syntax makes it possible to make scatterplots, line charts, histograms, and bar charts, among other tools for visualizing data.
- Caret: The caret library in R is used a lot for machine learning. It has many algorithms for grouping, sorting, and reducing the number of dimensions. It also has tools for evaluating and choosing models.
- Shiny: R’s shiny framework is ideal for creating web applications. Data analysts and scientists can use this platform to create dashboards, visualizations, and dynamic data tables.
Conclusion
In conclusion, both proprietary and open-source data analytics tools for beginners and researchers are available. Starting with user-friendly tools such as Excel or Google Sheets is recommended for beginners before moving on to more advanced tools such as Python or R. For researchers, various tools are available depending on the specific research needs, including data manipulation, visualization, and machine learning.
Tableau, Power BI, QlikView, Google Analytics, and SAS are some of the best tools for analyzing data. You can do many things with these technologies: analyze data, make data easy to understand, and make reports.
Also, many data analysts and researchers use open-source data analysis tools like Pandas, NumPy, Matplotlib, Scikit-learn, TensorFlow, Keras, PySpark, RStudio, ggplot2, dplyr, tidy, caret, shine, and data. Table. The active user community for these products gives help and instructions and offers a cheaper and more flexible way to analyze data.
When making a final choice, it’s also important to consider how easy it is to use, how scalable it is, and what kind of support it has. Whether you are a beginner or a seasoned data analyst, you can use various tools to help you evaluate your data and determine its meaning.