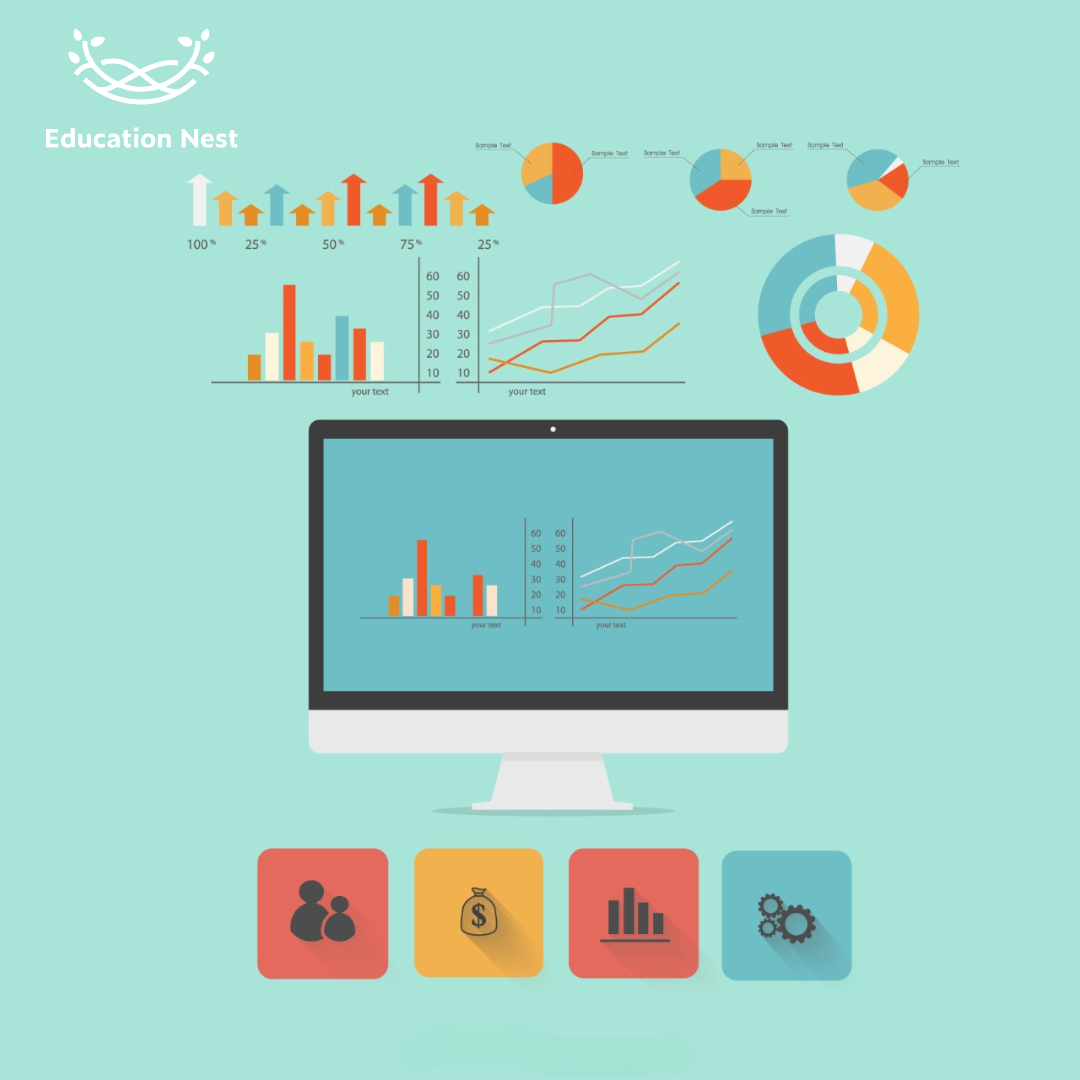
Are you looking to enhance your data visualization skills with Power BI Heatmaps? Look no further than this Ultimate Guide! In this blog, we’ll cover everything you need to know about Power BI Heatmaps, including how to create them, customize them, and analyze your data like a pro. Whether you’re a beginner or an expert, you’ll find valuable tips and tricks to take your data analysis to the next level. So, what are you waiting for? Let’s dive in!
A heatmap is a graphical representation of data that shows the relative values of a group of data points using color-coded cells. Heatmaps are frequently used to display vast and complicated data sets in an easy-to-understand and analyzed format.
Heatmaps are a standard visualization in Power BI dashboards for mapping analysis and displaying geospatial data. This article will explain these maps, how to utilize them, and how to create a desktop Power BI heatmap.
What are Power BI Heatmaps?
Each data point in a heatmap is represented by a cell whose color indicates how significant the point is. Heatmaps, which function best with multidimensional data, are frequently used to find patterns, trends, and linkages in large datasets.
Heatmaps are frequently used in business, finance, healthcare, and research, among others, to make data-driven decisions and convey ideas to stakeholders.
What is the color in the Power BI heatmap?
A color gradient in power bi heatmap is typically used to convert data values into a range of colors, with deeper hues representing higher values and lighter hues representing lower values. Each row and column represent a different category or variable; the cells are organized in a grid or matrix.
Features of Power BI Heatmaps
The following Power BI heatmap functions are available:
Data Aggregation: The grid in Power BI allows users to organize data by month, quarter, or year based on their measure and dimension(s).
Color Mapping: It allows users to add colors to integers or groups. After Power BI provides a color scale, the user can change the scale and style.
Tooltips: It shows when a user moves their mouse over a cell. The tooltip can be customized to display any meaningful measure, percentage, or trend.
Conditional Styling: In Power BI, conditional styling allows users to highlight cells or rows based on high or low values, trends, or data points that do not fit the pattern.
You Must Like: Unlock Your Potential: DevOps Interview Questions That Will Challenge You
How to build visual Power BI Heatmaps?
A specific collection of numbers is required to create a heat map.
Let’s start with a simple piece of data because this is our first time creating a heat map. You can either copy the data and paste it directly into Power BI or copy it to an Excel file and then import it as a reference to the Excel file into Power BI. You can also get the Excel file in this example by clicking the link below.
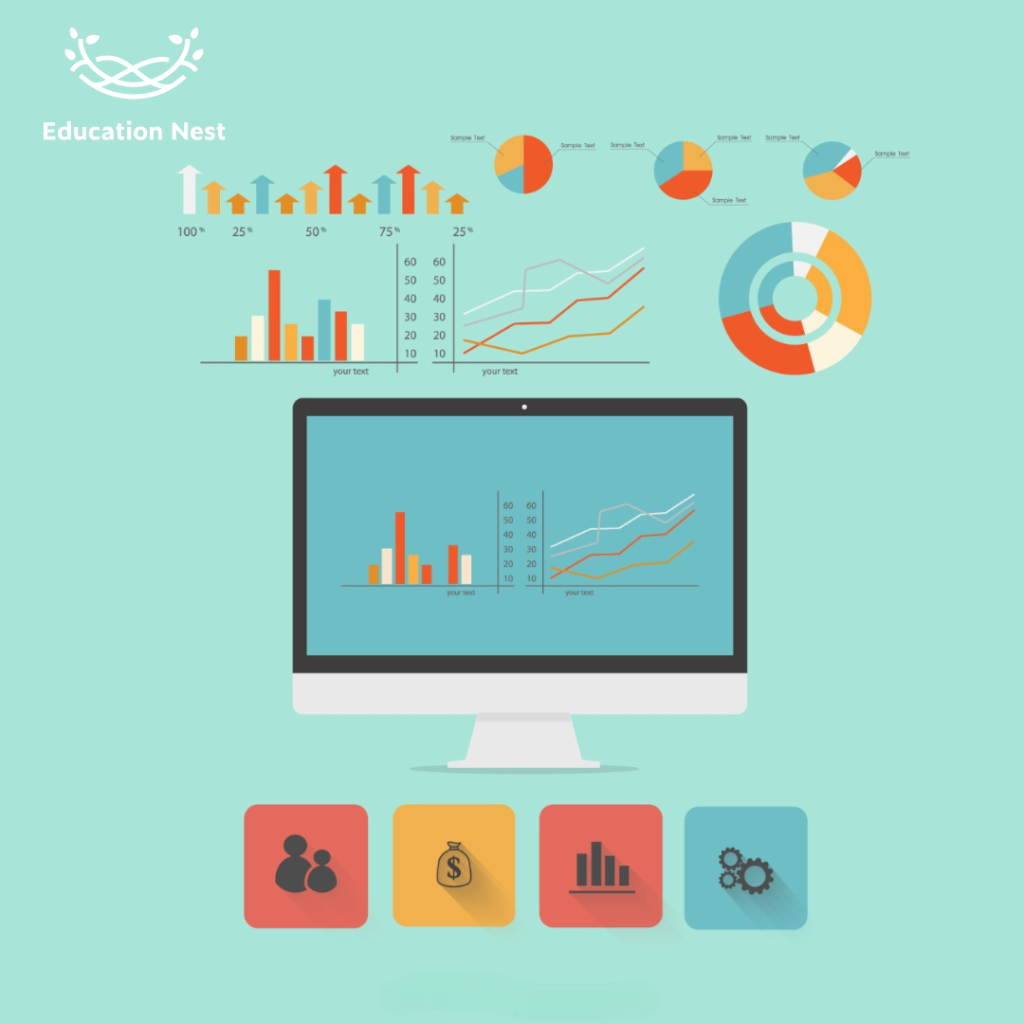
The data has been submitted directly to Power BI.
After that, we can create a heat map, but no ready-to-use show exists.
To create your unique visualization, follow these steps:
- First, navigate to the “Report” view.
- Navigate to the “Visualizations” panel, then click “Import from the marketplace,” followed by “Import a custom visual” (three dots at the bottom).
- If you already have a Power BI account, it will redirect you to the page where you can locate custom images. You will be asked to sign in if you still need to.
- Sign in with your company’s email address. You will be directed to the site.
- Enter “heat map” in the search field and press “Search.” There will be a list of related search results.
- Now press the “Add” button. This new image is part of the “Visualizations” image series.
- Click on the new image. We’ll have a blank “heat map” ready.
- You can drag and drop the “City” column into the “Location (ID)” field and the “Sales” column into the “Value” field using this image.
- Our “Heat Map” is now ready for usage.
- Go to the “Format” option and select “Heat map.”
Conclusion
The heatmap power BI is an effective tool for displaying patterns, trends, and outliers in massive datasets. This strategy allows users to easily identify patterns, trends, and outliers.