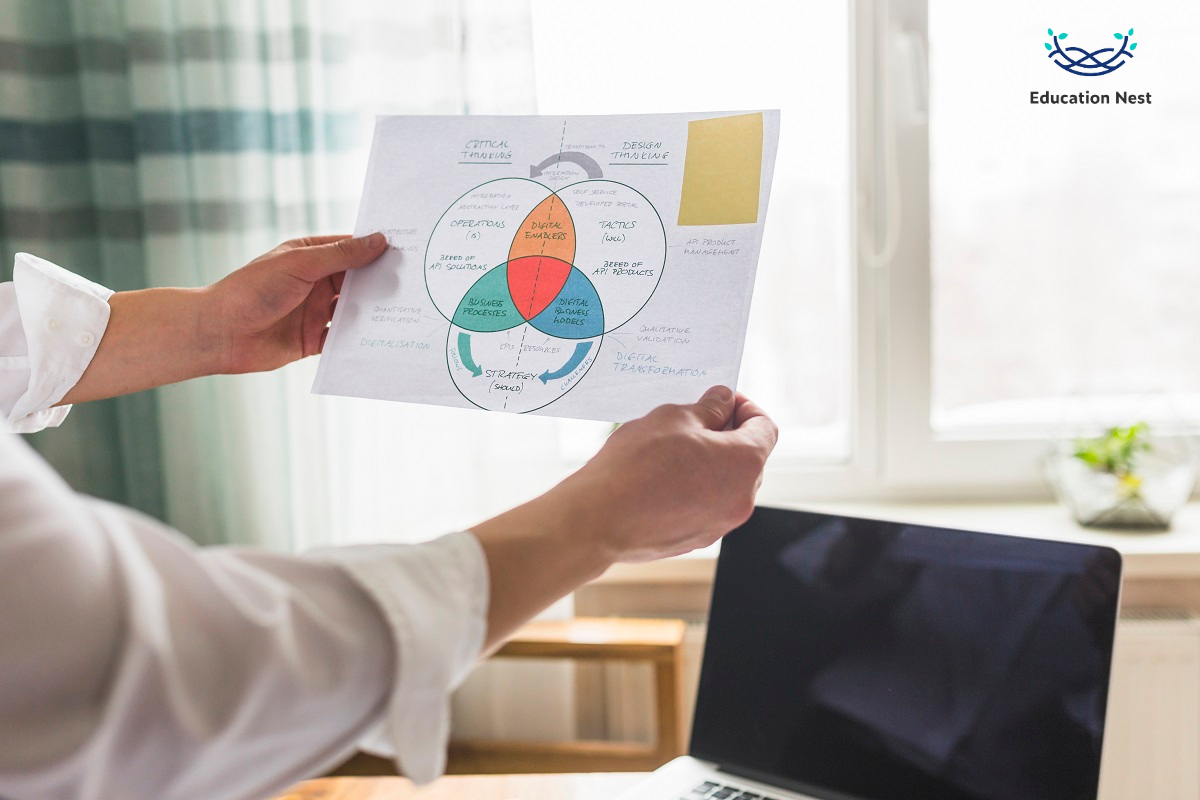
Data analysis is a systematic process that entails obtaining, cleaning, transforming, and analyzing data to develop relevant findings and assist decision-making. The data analysis life cycle is a well-organized structure for data analysis initiatives. This ensures the process is well-planned, correctly executed, and yields dependable outcomes.
This post examines the significant stages of the data analysis life cycle and discusses the best strategies to complete each step.
Data Analysis Life Cycle
Outline the Objectives and Questions
The first phase in the data analysis life cycle is to ensure that the study’s objectives and questions are clear. To do so, you must first understand the problem or chance at hand and the information you seek from the data. The subsequent phases in the data analysis process will help you attain your goals by making your goals and queries explicit.
Obtaining and Tidying up Information
After establishing the objectives and questions, the next stage is to collect the necessary data. This may necessitate obtaining data from various sources, including databases, files, and APIs. Ensuring that the data obtained is correct, thorough, and relevant to the study is critical. Cleaning the data is a crucial aspect of this procedure. This is where you search for and correct issues such as missing numbers, outliers, or inconsistencies in data collecting. Proper data cleansing ensures that the data used for further study is accurate and high-quality.
Data Exploration and Data Analysis
When you have clean data, you may go to the next step: exploration and analysis. Various statistical methodologies, visualization tools, and exploratory data analysis techniques are utilized at this step to generate ideas from the data. Data mining, descriptive statistics, and data visualization technologies can all be employed to uncover patterns, correlations, and trends in data. This procedure allows you to find out the data and provide some initial suggestions.
Making Models and Testing Hypotheses with Data
You create a data or statistical model at this level to test your hypotheses and make predictions. Regression analysis, machine learning algorithms, or other statistical methods may be utilized depending on the type of analysis to create models that explain the relationships between variables and generate predictions or group them. Hypothesis testing is also used to determine whether specific claims or hypotheses are correct or incorrect depending on the evidence.
Data Analysis Life Cycle | Interpretation and Visualization
It is critical to understand the results of designing and testing the data model. This entails dissecting the outputs of the models, determining how well they function, and making decisions based on the findings. The results can be displayed using data visualization tools such as charts, graphs, and dashboards. This makes it easier for stakeholders to comprehend and act on the investigation findings.
You Must Read: Hyperion Fundamentals for Financial Management
Data Analysis Life Cycle Help in Making Decisions and Communicating
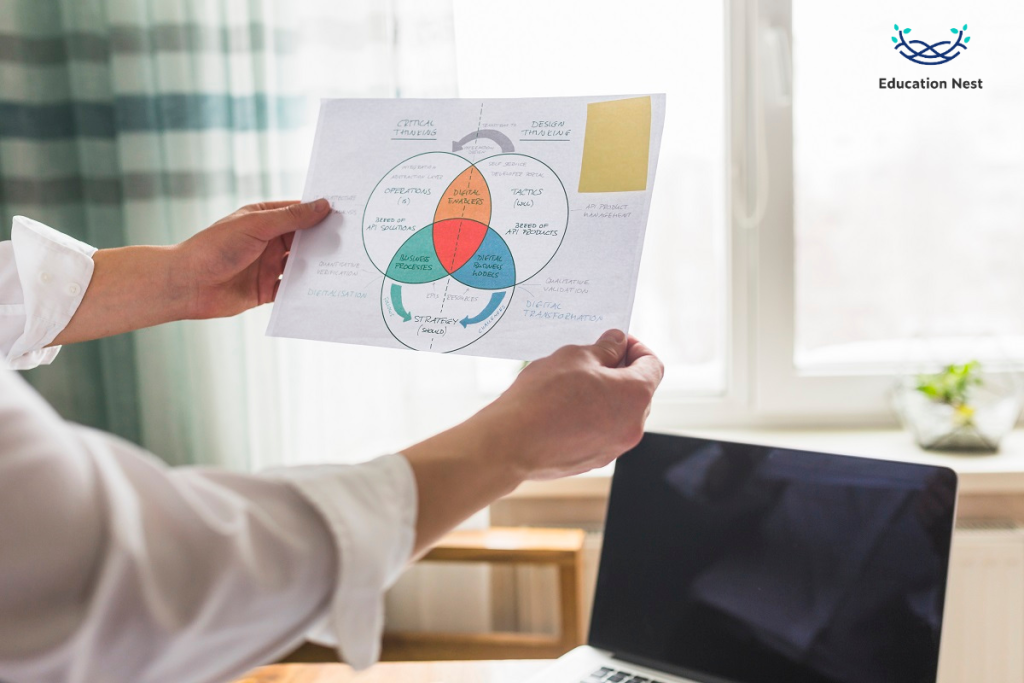
The final step in the data analysis life cycle is informing individuals about the findings and lessons learned. This entails creating materials such as reports, presentations, or visualizations that summarize the study findings in a few phrases and recommend applications for them. The message must be personalized to the audience for them to grasp it and use it to decide. Collaboration and participation from stakeholders are required to ensure that insights are used correctly to generate well-informed decisions and actions.
Conclusion
The data analysis life cycle enables data-driven decisions to be made in a planned manner. By following a well-defined process that includes setting goals, collecting and cleaning data, exploring and analyzing the data, modeling and testing hypotheses, interpreting the results, and effectively communicating the findings, organizations can use the power of data to drive innovation, improve operations, and achieve their goals.